Learn how a leading financial services company increased credit card spend by 15-20% with AI and machine learning. Read this BFSI case study to see how AI in marketing is transforming customer engagement and revenue growth.
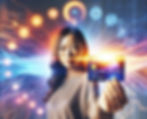
INTRO AND CLIENT BACKGROUND
Our client is a tech-forward financial services company providing simple, personalized payment, lending and saving solutions.
With a robust portfolio including private label credit cards, loyalty programs, and direct marketing, the client's success is deeply rooted in the capture and analysis of transaction-rich data.
Their business model revolves around using customer insights to cultivate meaningful brand-customer relationships, positioning them as a leader in the industry.
BUSINESS GOALS & CHALLENGES
The primary objective was to construct a powerful share of wallet model, a transformative tool for comparing Private Label Credit Card (PLCC) spend to total brand spend.
This innovative model aimed to spotlight customers not fully optimizing their card usage in comparison to other payment methods.
The strategic implementation of this model would facilitate targeted campaigns, focusing on existing customers poised to increase their spend using the PLCC card within the next twelve months
VALUE DELIVERED: WHAT WAS THE BUSINESS IMPACT?
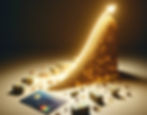
The innovative solutions implemented led to tangible benefits across various stakeholders:
Increased Card Spend by 15-20% - The model enabled the identification of customers likely to increase their spend using the PLCC card. By strategically targeting this high-potential audience, the solution contributed to a remarkable 15-20% increase in PLCC card spend.
Enhanced Marketing Strategies - Our solution allowed resources to be focused on the audiences most likely to drive card spend. The optimization of AI marketing in credit card segment not only elevated overall revenue but also fine-tuned campaigns, marking a significant improvement in marketing investment efficiency.
Strategic Decision-Making - The insights gleaned from the model now inform a spectrum of decisions, ranging from promotional strategies to the structuring of rewards programs.
TECH STACK

CONCLUSION
This case study underscores the power of AI and machine learning in reshaping marketing dynamics within the BFSI sector.
By leveraging data-driven insights, our client not only enhanced customer engagement but also witnessed tangible improvements in card spend and overall revenue.
The success story stands as a testament to the transformative potential of innovative technologies in driving strategic marketing decisions in the financial services domain.
We are here to help you improve your business decisions and seize opportunities. Reach out today to get a free consultation! - info@maticsanalytics.com | www.maticsanalytics.com/book-online